Performing the Luria-Delbruck experiment using rifampicin resistance to model mutational events
by Delaney J.W. Ragsdale
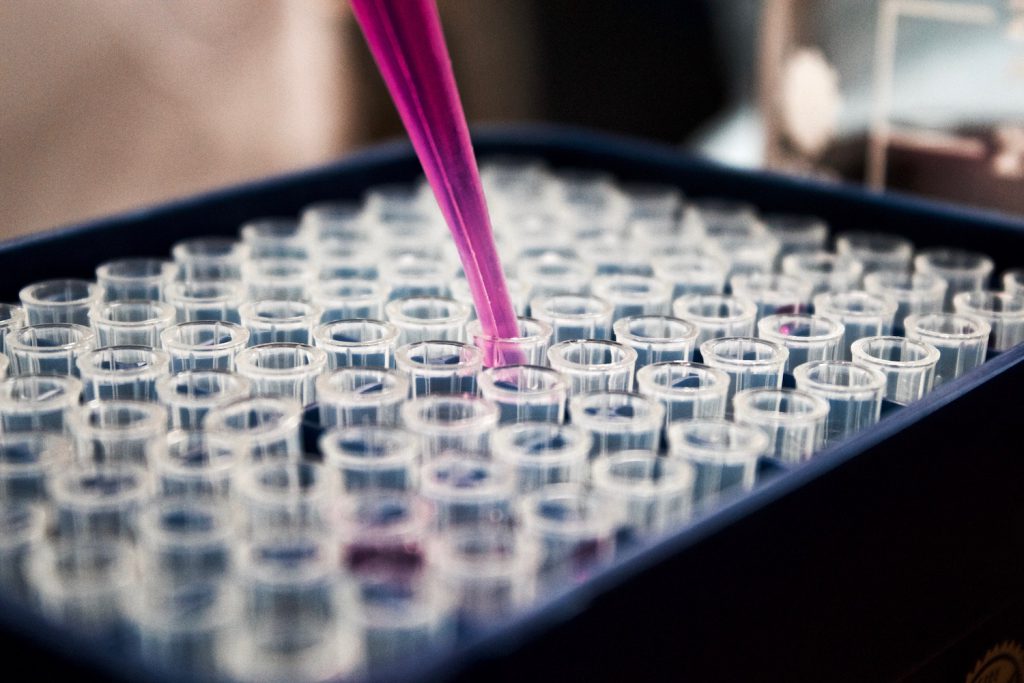
Once thought to be mutually exclusive, the competing ideas of Lamarckism and Darwinism attempt to describe the mechanism behind evolution and define the origin of mutations. To determine whether adaptive phenotypes arise due to directed mutation (Lamarckian) or random mutation (Darwinian), we performed the Luria-Delbruck experiment using rifampicin resistance to model mutational events in Escherichia coli. Across replicate populations, we observed a scattered distribution of rifampicin resistant colonies with a low mean, very high variance, and high standard deviation due to the high frequency of low rifR numbers and a low frequency of high rifR numbers. These results correspond to the expected results seen in the random mutation model and suggest that mutations arise before antibiotic exposure.
Keywords: Darwinism, random mutation, Lamarckism, directed mutation, antibiotic resistance
Introduction
The theory of evolution has existed for centuries but continues to stump scientists in their efforts to define the agent and driver of selection. The once-dominating theory of Lamarckian inheritance suggests that identical organisms acquire heritable characteristics during their lifetime to adapt to changing conditions or address new needs of the individual. Darwinism, on the other hand, describes a process where fluctuating environmental conditions act on pre-existing variation within a species, conferring natural selection in favor of beneficial variants1. Darwin’s theory did not address how beneficial traits are preserved across future generations, which remained unknown until discovery of Mendelian genetics. This model states that genes encode phenotypes, and alleles segregate independently into offspring. By applying the Mendelian idea of segregation to the Darwinian concept of natural selection, mutations were established as the driver of phenotypic variation, with genes as the vehicle of evolution. Yet, even after the evolutionary role of mutations was defined, the origin of these mutations remained unknown2.
The chromosome theory, which was widely accepted in the early 1900s, identified chromosomes as the inherited genetic material and was used to explain inheritance in highly complex organisms, such as humans. However, many scientists concluded that bacteria did not contain genetic material and fell back on the Lamarckian theory to explain the non-Mendelian inheritance patterns often observed in bacteria2. It appeared as though bacteria could pass on acquired characteristics, such as antibiotic resistance, to future generations after initial exposure to the compound3. In an effort to investigate the genetic mechanism underlying this unexpected pattern of phenotypic expression, Salvador Luria and Max Delbruck demonstrated how mutations arise in the absence of selection rather than responding to the selection itself4.
By investigating possible causative factors behind heritable variation, the Luria-Delbruck experiment eloquently proved that pre-existing genetic mutations in bacteria undergo selection when environmental pressure is applied4. Therefore, it also rejects the Lamarckian concept that states environmental conditions cause cells to change in an attempt to survive. This experiment not only laid the foundation for bacterial genetics, but also established fundamental mechanistic ideas behind evolution that are still supported today. While the original experiment studied bacterial resistance to bacteriophage4, our class will be performing this experiment using rifampicin resistance to model mutation events in Escherichia coli.
To determine whether resistant cells arise due to directed mutation or random mutation, we will plate equal amounts of wildtype cells from the same starting culture on plates containing rifampicin. We will then compare the distribution of rifampicin-resistant colonies to both the directed and random mutation models to see which mutation hypothesis best represents our data. If resistant cells arise in response to the antibiotic being applied (directed mutation hypothesis), the results should show a low variance between replicate populations. All sensitive cells would have the same small probability of mutating into a resistant state. If resistant cells arise before antibiotic application (random mutation hypothesis), then there would be a high variance between replicates. Most replicates would have a low number of resistant cells while fewer replicates would possess a large number. Also, if the mutation occurs early in population growth, we will see a large number of resistant cells in the replicate since every subsequent generation of the mutant lineage will inherent rifampicin resistance4.
Methods
Escherichia coli as a study system
Because the characteristic of asexual splitting is vital to the Luria-Delbruck experiment, Escherichia coli serves as the perfect candidate for studying random versus directed mutations. As E. coli undergoes clonal expansion, each mutation gets passed along to identical daughter cells and will be present throughout an entire individual colony. In this experiment, mutations in these rifampicin-sensitive (rifS) bacteria were identified by their newfound resistance to the antibiotic.
Identifying mutation events
To ensure most cells share a common ancestor, we started 32 E. coli cultures with a low starting concentration of 10 colony forming units (CFU). This concentration was determined by titering the initial culture and calculating the volume needed for a 500,000-fold dilution. All cultures were grown in liquid media without antibiotics. After incubation, cultures 1-30 were examined for cloudiness and labeled as viable if growth was observed. Only one culture had no indications of growth probably due to unsuccessful inoculation and was removed from the experiment. Viable cultures serving as replicate populations (1-29) were plated onto agar containing rifampicin to select for mutants. Only rifampicin-resistant (rifR) cells will survive and form colonies. The number of CFUs will represent how many rifR cells were present in each population at the time of plating.
To confirm the actual number of sensitive cells subcultured (N0), the remaining two cultures (31-32) were used to determine the approximate titer of each replicate population. Both serial dilutions were plated onto LB plates and the number of CFUs were counted. The mean of these two titers was used to calculate the average number of cells in each culture (N). Then, the fraction of rifampicin plates with zero colonies (f) was determined. Finally, the mutation rate of rifampicin resistance per bacterial doubling was calculated by entering these values into the equation below:
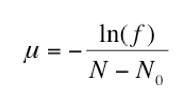
Results
Replicate populations displayed a varying and scattered number of rifR colonies (Fig. 1). The majority of antibiotic plates had very few to zero colonies due to the rare occurrence of mutations, however a low frequency of “jackpots” consisting of more than 20 resistant colonies was observed. Thus, the wide range in CFUs does not follow a normal distribution and is skewed towards the lower numbers with only a few instances of high numbers.
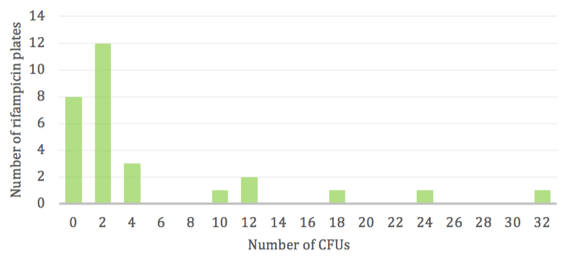
Table 1: Summary of the number of rifR colonies growing on each plate throughout the class. Groups (G) are listed horizontally across the top and plates are identified by numbers listed vertically on the left. Our data is listed under ‘G1’. “Jackpots” are labeled in bold.
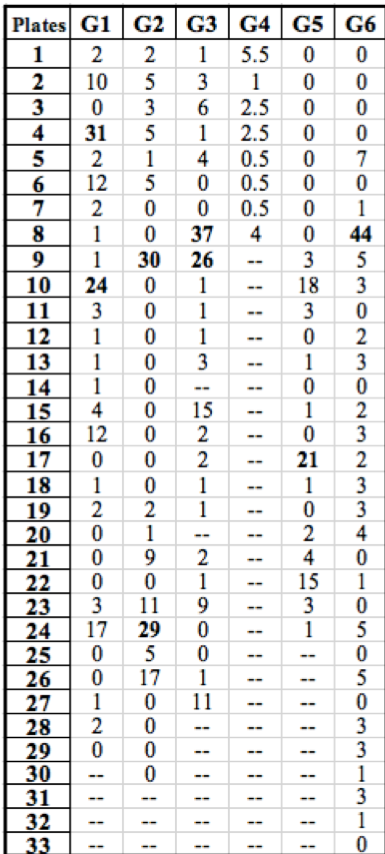
Our data is similar to the collective results from the rest of the class (Table 1). Of the 30 initial cultures that were simultaneously started, 29 successfully grew during incubation and were used in our experiment. Other groups had similar results and plated around the same number of replicate populations. As was the case with our data, the other experiments resulted in a high frequency of low colony numbers with a few “jackpots” containing a much greater occurrence of rifR colonies. However, the results from Group 4 do not seem to follow this trend due to the low number of successfully grown replicate populations in liquid media.
After using Microsoft Excel to calculate the mean, average, and standard deviation of each group’s results (Table 2), we found that the variance of each experiment was often more than ten times greater than the mean taken from the same set of data, except for Group 4. The large amount of low rifR frequency seems to outweigh the few “jackpots” which is represented in the generally small mean value of each group. The variance accounts for these “jackpots” which drastically increases its value in comparison to the mean value. The relatively high standard deviations calculated for each data set describes the large range of rifR colonies per individual plate.
Table 2: Summary of the mean, variance, and standard deviation calculated with class data for six groups. Groups are listed horizontally across the top with their respective calculations below. Units are rifR colonies per plate.

To confirm the concentration of sensitive cells transferred from the initial culture, the titers of replicate populations 31-32 were calculated by plating both dilution series onto regular agar. After incubation, we found that these two sets of plates were unusable due to Neurospora contamination . In order to calculate the mutation rate, we used titer data from another group since every culture was made from the same initial E. coli culture. Using these values, the rifampicin-resistance mutation rate (μ) equals 3.03 10-9 mutations per doubling time.
Discussion
To determine how these mutations arose, we compared the directed and random mutation models to our experimental results to reveal which mutation hypothesis best represents our findings. Our data exhibited a very uneven distribution in the number of resistant cells per replicate population. While most rifampicin plates had very few colonies, the presence of “jackpots” dramatically widened this range. A high colony count indicates an early occurrence of mutational events during population growth. As the majority of replicates had very few or no colonies, it is likely that the probability for mutation is low. Mutational events are most likely to occur in large bacterial populations due to increased odds by simply having more cells that could potentially incur mutation. These results are consistent with class data and support the random mutation model, indicating that resistant mutations arise independently of antibiotic application4.
To further differentiate between the two mutation hypotheses, the mean, variance, and standard deviations were calculated for the class-wide dataset4. The high frequency of low rifR colonies decreases the mean value. The variance, on the other hand, is extremely large due to the occurrence of “jackpot” replicates. Five out of the six datasets have variances that are well above 10 times the average, indicating that most values are substantially different from the mean value. Standard deviation is also very high with five out of the six datasets resulting in a standard deviation nearly twice as large as the average. Two of the five standard deviations are almost double that of the mean value suggesting widely distributed data points. The mean, variance, and standard deviation results correspond with the expected outcome of the random mutation model4.
If our data reflected the directed mutation hypothesis, we would have observed a relatively even distribution with a small variance. The calculated variance would be similar to the mean values and standard deviation would be low. These results would have indicated acquired resistance in response to antibiotic exposure4. In the absence of this pattern, our experimental data rejects both the model of directed mutation and that of Lamarckian inheritance.
Despite our results suggesting that evolution occurs via the random mutation hypothesis, a recent re-emergence of Lamarckian ideas may argue that not all mutations are random. Many well-studied biological systems, such as CRISPR-Cas9, piRNA, and horizontal gene transfer (HGT), exemplify the modern understanding of Lamarckian mechanisms5. The CRISPR-Cas9 system, traditionally utilized for cell-immunity, inserts fragments of bacteriophage or plasmid genes into CRISPR cassettes within the genome to be used as guide molecules for phage destruction in the future. Transposon-derived piRNAs form rapidly proliferating clusters that provide defense against transposable elements in the germ lines of all animals. After being exposed to antibiotics, many bacterial species may undergo HGT in hopes of acquiring antibiotic resistance genes from extracellular DNA fragments or plasmids. From a generalized mechanistic view, these pathways employ environmental signals to target specific genes for directed mutation. The resulting modifications, such as phage immunity, defense against mobile elements, and antibiotic resistance seen in these examples, are heritable and adaptive to the original conditions that initiated genomic targeting3.
Examples of quasi-Lamarckian phenomena, such as stress-induced mutagenesis, utilize environmental signals to cause phenotypic variation while diverging from the strict Lamarckian guidelines. The mutagenic “SOS repair” pathway, also utilized by our study system E. coli, is activated as an effort to survive by generating non-random mutations near double-strand breaks in the genome. This type of regulated mutagenesis is thought to allow the coordinated evolution of clustered, functionally linked genes, where beneficial mutations emerge while limiting the damage to other parts of the genome. Stress-induced mutagenesis pathways have been discovered in bacteria, yeast, and animal cells, suggesting that this mechanism of adaptive evolvability is general across the entire range of life3. While this pathway employs environmental signals such as stress to initiate heritable modifications, these mutations do not guarantee adaptability. Instead, the induced variation is selected upon via environmental conditions where beneficial mutations can then become fixed in a population. This has also been observed in epigenetic markers, where environmental signals are used to direct DNA modifications, such as methylation or acetylation. Addition of these groups confer hereditable, phenotypic adaptability through changes in gene expression without alteration to the DNA sequence3.
These quasi-Lamarckian biological processes seem to combine different aspects of Darwinism and Lamarckism, indicating that the two evolutionary hypotheses may not be mutually exclusive. This combination may help with the dissolution of a perceived conflict between Darwinism and Lamarckism. Both may have a role in adaptive evolution under different circumstances. Further study into the coexistence of both types of mechanisms within E. coli is needed to assess relative importance to evolutionary adaptation. The version of the Luria-Delbruck experiment that we performed depends on the mutual exclusivity of the directed and random mutation hypotheses. This limits interpretation of the results to either one hypothesis or the other, however, the possibility that both models may coexist should be taken into account. Future studies may find it insightful to quantify the frequencies of mutations either occurring randomly or via directed mutagenesis.
Literature Cited
- de Meeûs, T., & Prugnolle, F. Clonal Evolution. Genetics and Evolution of Infectious Diseases: Second Edition. (2017).
- Robbins, R. J. Introduction to the Demonstration of True Genetic Mutation in Bacteria. ESP. iii-xi. (2001).
- Koonin, E. V., & Wolf, Y. I. Is evolution Darwinian or/and Lamarckian?. Biology direct. 4:42. (2009).
- Luria, S.E. & Delbrück, M. Mutations of bacteria from virus sensitivity to virus resistance. Genetics. 28: 491-511. (1943).
- Poole, A. M. Horizontal gene transfer and the earliest stages of the evolution of life. Research in Microbiology. 160(7):473-480. (2009).
Acknowledgements
Delaney would like to thank Dr. Jonathan Eggenschwiler, Dr. Andrea Sweigart, Margot Popecki, and Shaugnessy McCann for guiding and providing expert advice on this research project. She would also like to thank Brittany Borzillo for helping with experiments as well as Dr. Lindsey Harding for the refinement of this piece. Finally, she would like to thank her professor and mentor, Dr. Vincent Starai, for his generosity and invaluable insights.